This blog was originally published on ISACA Now.
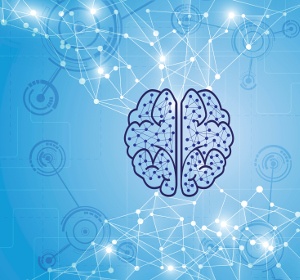
In many spheres of employment, the application of artificial intelligence (AI) technology is creating a growing fear. Kevin Maney of Newsweek vividly summarized the pending transformation of employment and the concerns it raises in his recent article "How artificial intelligence and robots will radically transform the economy."
In the Information Security (InfoSec) community, AI is commonly seen as a savior—an application of technology that will allow businesses to more rapidly identify and mitigate threats, without having to add more humans. That human factor is commonly seen as a business inhibitor as the necessary skills and experience are both costly and difficult to obtain.
As a consequence, over the last few years, many vendors have re-engineered and re-branded their products as employing AI—both as a hat-tip to their customer’s growing frustrations that combating every new threat requires additional personnel to look after the tools and products being sold to them, and as a differentiator amongst “legacy” approaches to dealing with the threats that persist despite two decades of detection innovation.
The rebranding, remarketing, and inclusion of various data science buzzwords—machine intelligence, machine learning, big data, data lakes, unsupervised learning—into product sales pitches and collateral have made it appear that security automation is the same as AI security.
We are still at the very early days of the AI revolution. Product and service vendors are advancing their v1.0 AI engines and are predominantly focused on solving two challenges—sifting through an expanding trove of threat data for actionable nuggets and replicating the most common and basic human security analyst functions.
Neither challenge is particularly demanding of an AI platform. Statistical approaches to anomaly detection, data clustering and labeling processes meet all the criteria for the first security challenge, while “expert system” approaches of the 1970s and 1980s tend to be adequate for most of the second challenge. What’s changed is volume of data that decisions must be based upon and the advances in learning systems.
What is confusing many security technology buyers at the moment lies with the inclusion of AI buzzwords around products and services that are essentially delivering "automation."
Many of the heavily marketed value propositions have to do with automating many of the manual tasks that a threat analyst or incident responder would undertake in their day-to-day activities, such as sifting through critical alerts, correlating them with other lesser alerts and log entries, pulling packet captures (PCAPs) and host activity logs, overlaying external threat intelligence and data feeds, and presenting an analytics package for a human analyst to determine the next actions. All these linked actions can of course be easily automated using scripting languages if the organization was so inclined.
The automation of security event handling doesn’t require AI—at least not the kind or level of AI that we anticipate will cause a global economic and employment transformation.
The AI v1.0 being employed in many of today’s products may be best thought of as assembly-line robots—replicating repeated mechanical tasks, not necessarily requiring any "intelligence" as such. That automation obviously brings efficiencies and consistency to incident investigation and response—but by itself isn’t yet having an impact on an organization’s need to employ skilled human analysts.
As organizations get more comfortable sharing and collectively pooling data, the security community can anticipate the advancement and incorporation of better learning systems – driving down an incremental AI v1.1 path—in which process automation efficiently learns the quirks, actions and common decisions of the environment within which it is operating. One example would be assessing an analytics package that was automatically compiled by determining similarities with previously generated and actioned packages, assigning a prioritization and routing to the correct human responder. It may sound like a small but logical process of automation, but requires another level and class of math, and “intelligence” to learn and tune an expert decision making process.
In my mind, Security AI v2.0 lies in an intelligence engine that not only dynamically learns through observing the repeated classification of threats and their corresponding actions, but is able to correctly identify suspicious behaviors it has never seen before, determine the context of the situation and initiate the most appropriate actions on behalf of the organization.
That might include the ability to not just identify that a new host has been added to the network and appears to be launching a port scan against the active directory server, but to predict whether the action may be part of a penetration test (pentest) by understanding the typical pentest delivery process, typical targets of past pentests and the regular cadence or scheduling of pentests within the organization. The engine could then arrive at an evidence-based conclusion, track down and alert the business owners of the suspected activity and, while waiting for confirmation, automatically adjust threat prevention rules and alerting thresholds to isolate the suspicious activity to minimize potential harm.
The success of security AI lies in determining actions based off incomplete and previously unclassified information—at which point the hard-to-retain "tier-one" security analyst roles will disappear like so many assembly-line jobs in the motor vehicle industry have in the past couple decades.